The fusion of AI with investment analysis is reshaping the financial industry. Recent studies from J.P. Morgan AI Research, the AI4 Finance Foundation, and Stanford University underscore this shift, showing how large investment funds are increasingly building custom AI platforms. These systems are designed to streamline deal sourcing, valuation, and equity research. One notable example, FinRobot, leverages large language models to automate and enhance traditional research workflows.
But what does this really mean for investors? How can AI tools be practically applied to your strategy—and do they actually improve returns? While the potential is huge, the technology is still evolving. Using AI in finance holds promise, but it’s not a guaranteed moneymaker just yet. That said, the field is full of intriguing developments worth exploring.
To dive deeper, we’ll explore three key areas where AI is actively shaping the investment landscape: custom in-house AI platforms for investment funds, multi-agent systems for equity research, and large language models for predictive stock ratings. While these tools are still maturing, early signs suggest they’re redefining what's possible in financial analysis.
In-House AI Platforms for Funds
In-house AI platforms are becoming increasingly prevalent among investment funds, offering tailored solutions for deal sourcing, data analysis, and decision-making processes. These bespoke platforms provide personalization, enhanced learning capabilities, and diverse use cases spanning multiple functions of the investment cycle. Smaller funds are now adopting AI technologies with the help of third-party providers, focusing on priority use cases such as deal sourcing and insights generation.
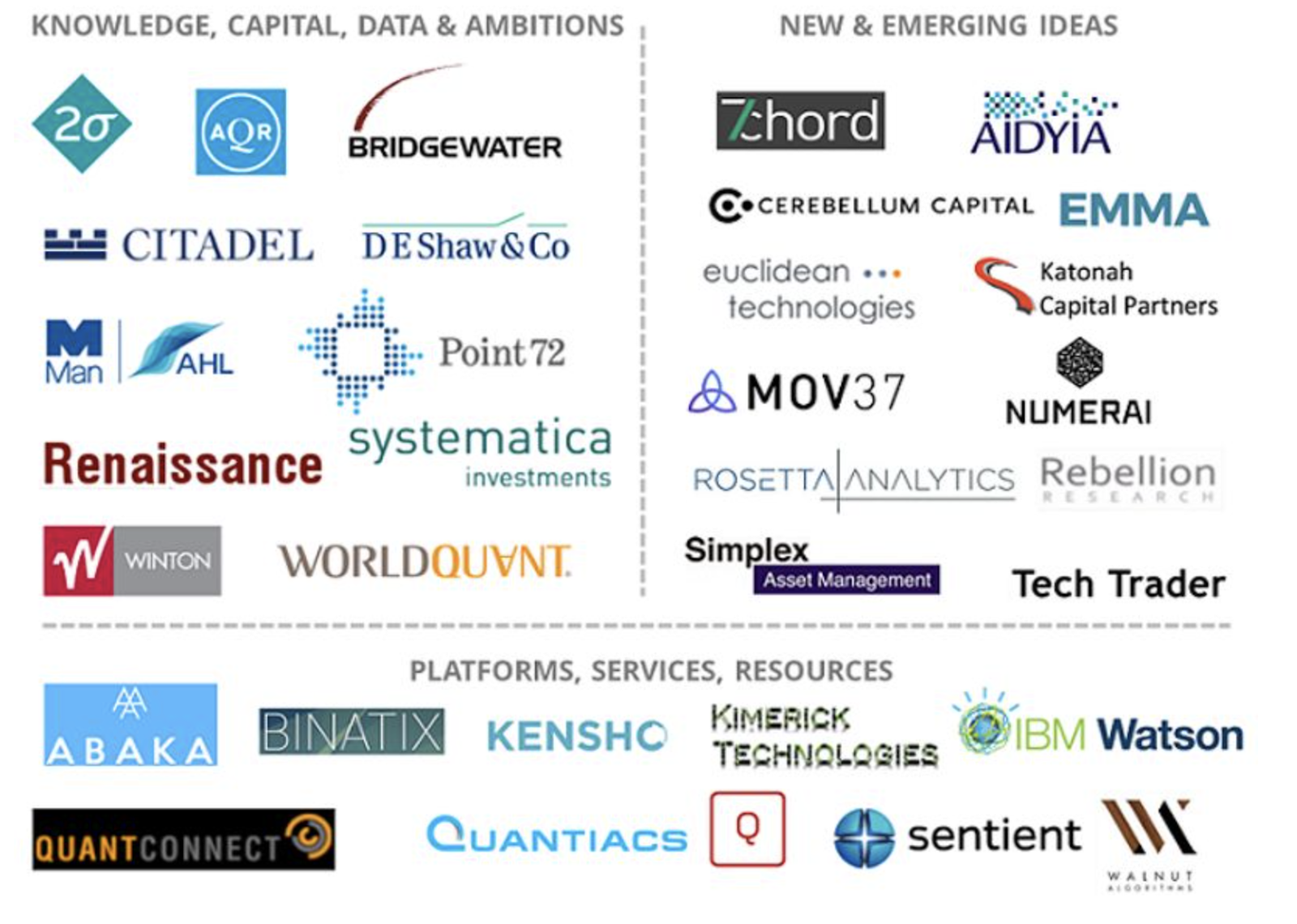
Key considerations for implementing in-house AI platforms by Stanford University include:
- Defining platform requirements and features, emphasizing adaptability and privacy measures
- Identifying high-return, low-risk use cases across the investment cycle
- Establishing robust data pipelines from public databases, proprietary fund data, and confidential asset information
- Selecting appropriate models and algorithms for specific investment strategies
These AI-driven tools are revolutionizing due diligence processes, leveraging machine learning and natural language processing to automate tasks like data analysis, risk assessment, and pattern recognition in vast amounts of financial documents. As a result, funds can make better decisions faster, with some quantitative investors reporting potential outperformance of 1.5% to 2% compared to traditional models.
Multi-Agent Systems in Equity Research
Multi-agent systems (MAS) are revolutionizing equity research by leveraging collective intelligence to tackle complex financial problems. These systems bring efficiency, adaptability, and automation to financial analysis, transforming traditional research methodologies.
Quick Overview of Multi-Agent Systems (MAS)
What is MAS?
Multi-Agent Systems (MAS) are an advanced framework in artificial intelligence where multiple autonomous agents interact, collaborate, and sometimes compete to achieve shared objectives or solve intricate problems. These agents function independently, yet their collective actions contribute to the system’s overarching goals.
Key Characteristics of MAS:
- Autonomy: Each agent operates independently without direct external control.
- Local Views: Agents possess limited knowledge about the global environment but rely on local data to perform tasks.
- Decentralization: Decision-making processes are distributed among agents, fostering flexibility and resilience.
- Adaptability: Agents can learn from interactions and adjust their behavior to dynamic environments.
A notable example of MAS in equity research is FinRobot, an open-source AI agent framework developed by the AI4 Finance Foundation,. FinRobot leverages a three-layer Chain of Thought (CoT) architecture, featuring specialized agents designed for specific tasks:
- Data-CoT: Processes and organizes data for financial analysis.
- Concept-CoT: Extracts insights and key concepts from processed data.
- Thesis-CoT: Synthesizes comprehensive research reports, replicating the thought process of human equity analysts.
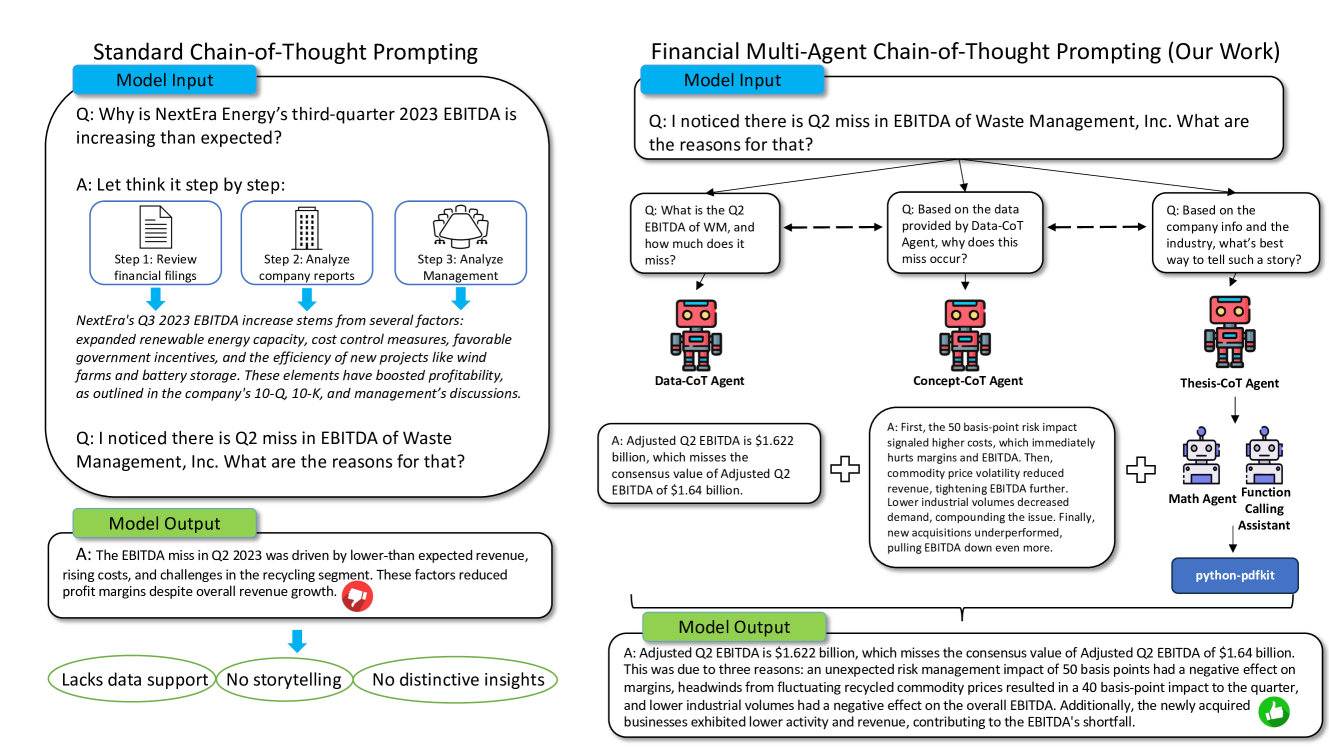
Key advantages of MAS in equity research include:
- Improved processing of vast, diverse datasets in real-time
- Enhanced adaptability to dynamic market conditions
- More robust decision-making through collective intelligence
- Potential to outperform both traditional algorithms and human experts
As financial markets become increasingly complex and data-driven, multi-agent systems like FinRobot are emerging as powerful analytical tools. FinRobot's expert review revealed high performance across accuracy (9.7/10), logical coherence (9.3/10), and storytelling (8/10), with reviewers praising comprehensive data coverage while suggesting improvements in narrative engagement and contextual storytelling. These advanced frameworks enable hedge funds and algorithmic trading firms to gain a competitive edge by delivering sophisticated, data-rich insights that bridge quantitative precision with qualitative understanding.
"Explore the AI-powered FinRobot framework on GitHub: AI4Finance-Foundation/FinRobot."
LLMs for Predictive Stock Ratings
Large Language Models (LLMs) are demonstrating significant potential in predicting stock performance and generating stock ratings, outperforming traditional methods when assessed by forward returns. Recent research by J.P. Morgan AI Research utilizing GPT-4-32k (v0613) on diverse datasets from January 2022 to June 2024 shows that incorporating financial fundamentals enhances rating accuracy. Interestingly, while integrating news data improves short-term performance, omitting it entirely can enhance long-term predictions by reducing bias.

The efficacy of LLMs in stock rating prediction varies across different models and investment universes. Decoder LLMs-based prediction models lead to stronger portfolios in larger investment universes, while results are less consistent in smaller ones. Among tested models, Mistral demonstrates robust performance across various universes. However, it's crucial to note that even state-of-the-art LLMs show limitations, with prediction accuracy ranging from 51.6% to 65.6% across 250 S&P 500 stocks. This underscores the inherent challenges in reliably predicting stock price movements, even with advanced AI tools.
Shaping Tomorrow: AI's Impact on Investment
The convergence of AI technologies in investment analysis is reshaping the financial landscape, with implications spanning from individual stock analysis to broader market strategies.

Key trends defining this transformation include:
As we synthesize the current state of AI in finance, several key trends and future directions emerge:
- Smarter AI Models: Advancements in LLMs and multi-agent systems are set to elevate market forecasting accuracy and adaptability.
- Ethics and Transparency: Ensuring fairness and clarity in AI-driven decisions is critical for trust and compliance.
- Human-AI Synergy: The future lies in blending AI's computational power with human intuition and expertise.
- Regulatory Evolution: Policymakers must adapt frameworks to foster innovation while protecting market integrity.
- Democratized Access: AI tools are now accessible to individual investors and smaller funds, leveling the playing field.
Practical Recommendations:
For Investors:
- Adopt AI-Driven Tools: Leverage cutting-edge AI platforms for deeper market insights, improved risk assessment, and a sharper competitive edge.
- Combine AI with Human Expertise: Balance the precision of AI with your intuition and experience to make well-rounded investment decisions.
- Stay Informed: Keep up with advancements in AI technologies to ensure you're utilizing the best tools available.
For Firms:
- Build AI Expertise: Invest in top AI talent to create innovative solutions tailored to your investment strategies.
- Strengthen Data Infrastructure: Ensure seamless data pipelines that can handle public, proprietary, and confidential information effectively.
- Foster a Growth Culture: Encourage continuous learning and adaptability to stay ahead in an ever-evolving technological landscape.
For Regulators:
- Develop Transparent Frameworks: Design policies that promote ethical AI use while safeguarding market integrity.
- Address Algorithmic Bias: Implement standards to reduce bias and enhance fairness in AI-driven decisions.
- Promote Interpretability: Require AI systems to explain their decision-making processes, building trust among stakeholders.
The future of investment lies at the intersection of human ingenuity and AI's transformative potential. The question is not whether to adopt AI—but how to maximize its promise for the journey ahead.
"AI is the compass guiding the future of investment—adapt, innovate, and thrive."