The Missing Piece in Conversational Recommenders
Conversational recommender systems have traditionally focused on two main tasks: recommending items accurately and generating coherent responses. Despite advancements in integrating knowledge graphs and pre-trained language models, these systems typically operate under the flawed assumption that all items in training datasets are optimal recommendations and that standard responses adequately engage users.
This assumption leads to a critical misalignment between system outputs and actual user needs. Conventional CRSs may fail to recognize when users express negative emotions about suggested items, and their responses often lack emotional engagement—resulting in interactions that feel mechanical rather than human-like.
As the authors pointedly observe, emotions play a crucial role in human decision-making. By capturing and responding to emotions expressed in user utterances, recommendation systems can achieve better preference modeling and create more engaging user experiences.
What Is Empathy in Recommender Systems?
The researchers define empathy within a CRS as "the system's capacity to capture and express emotions." This definition encompasses two essential capabilities:
1. Emotion detection - Understanding user emotions expressed through natural language
2. Emotion expression - Generating responses that convey appropriate emotions
Through these capabilities, an empathetic CRS can better distinguish and fulfill user needs during both recommendation and response generation phases.
The ECR Framework: Engineering Emotional Intelligence
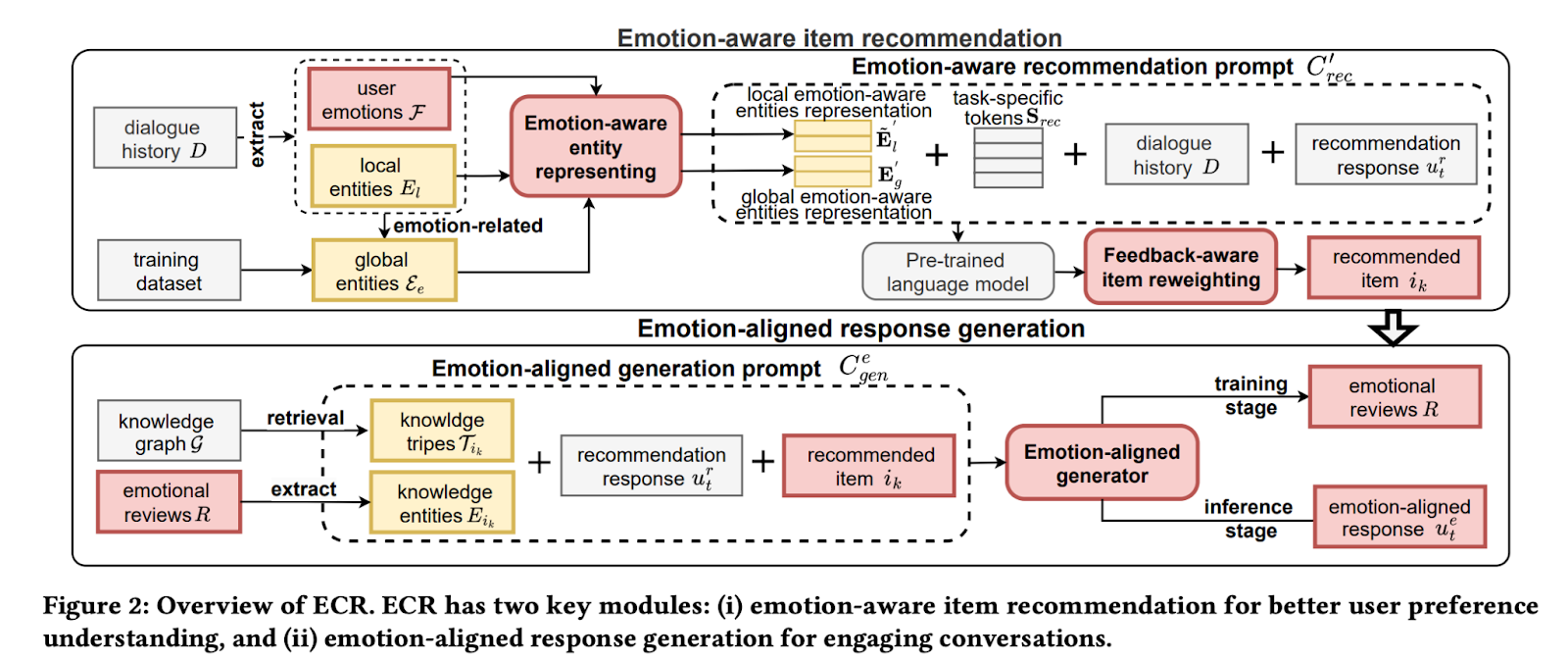
To address these challenges, the authors propose the Empathetic Conversational Recommender (ECR) framework with two primary modules:
Emotion-Aware Item Recommendation
This module integrates user emotions into the recommendation process through several innovative techniques:
1. Local emotion-aware entity representation: The system links emotions expressed by users to entities mentioned in the dialogue, creating emotion-aware representations of these local entities.
2. Global emotion-aware entity representation: Using collaborative knowledge from the training dataset, the system identifies global entities (items not mentioned in the current conversation) that relate to the user's emotional patterns.
3. Feedback-aware item reweighting: The framework implements a strategy that weighs recommendation candidates based on previous user feedback, helping to minimize the impact of incorrect labels in training datasets.
In essence, this module helps the system understand that when a user says "I hated that Shakespeare play," they're expressing a negative emotion toward Shakespeare that should influence future recommendations.
Emotion-Aligned Response Generation
The second module focuses on generating responses that express emotions, making interactions more natural and engaging:
1. Emotion-aligned generation prompts: The system retrieves relevant knowledge about recommended items from knowledge graphs and uses this information to create prompts that guide response generation.
2. Fine-tuned language models: The researchers fine-tune pre-trained language models on emotionally rich review data to generate responses that express appropriate emotions while maintaining factual accuracy.
This approach helps overcome the "emotional blandness" of standard CRS responses, replacing generic recommendations like "You might like Romeo and Juliet" with emotionally rich alternatives such as "I was completely moved by Romeo and Juliet! The way Shakespeare captures the intensity of young love really touched my heart."
Data Enrichment: Teaching Systems to Understand Emotions
A significant challenge in building empathetic systems is the lack of emotion-labeled training data. The researchers tackled this problem through clever data enlargement techniques:
1. They employed GPT-3.5-turbo to annotate user emotions in over 5,000 utterances from the ReDial dataset, using nine emotion labels including "like," "curious," "happy," "grateful," and "negative."
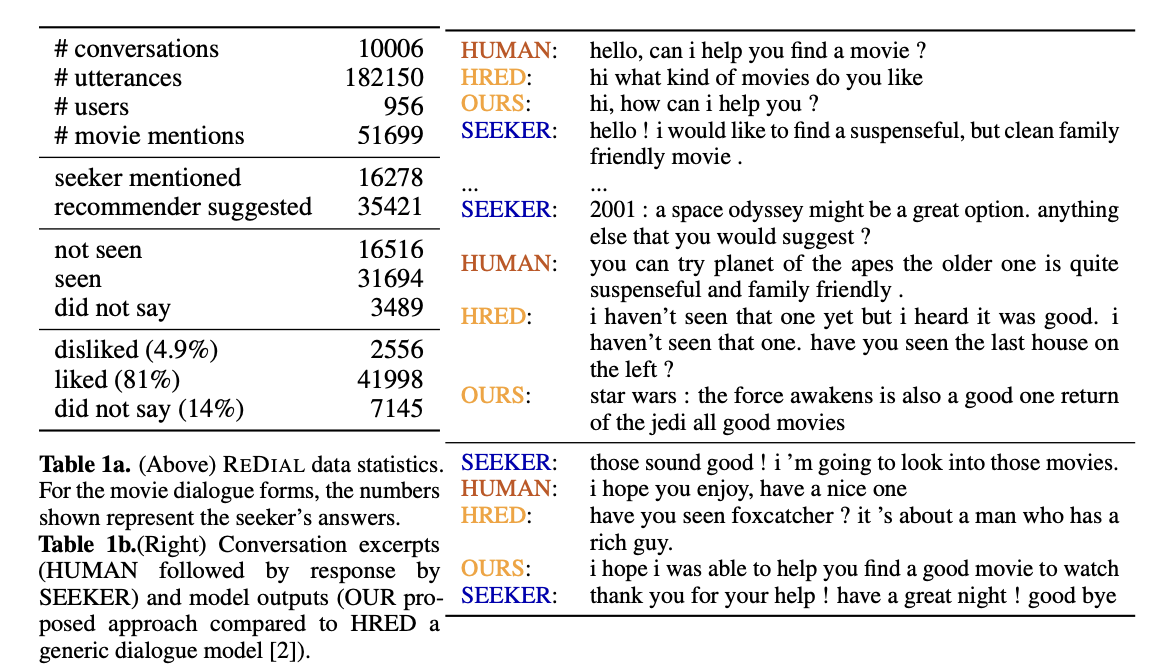
2. They fine-tuned a GPT-2 model on these annotations, achieving 87.75% recall accuracy, then used this model to label the entire dataset.
3. For generating emotional responses, they collected top-rated (10/10) movie reviews from IMDb that were rich in positive emotions, creating a database of emotionally expressive content.
This data enlargement approach provides valuable resources for training empathetic conversational systems, addressing the scarcity of emotion-labeled dialogue data.
Evaluation: Measuring Emotional Intelligence
The researchers introduced novel evaluation methods specifically designed to assess empathetic recommendation:
Objective Metrics
For recommendation accuracy, they used:
- Recall@n (R@n): Traditional metric for recommendation relevance
- Recall_True@n (RT@n): Only considers items that received positive user feedback
- Area Under the Curve (AUC): Assesses if items with positive feedback are ranked higher than those with negative feedback
Subjective Metrics
For response quality, they evaluated five dimensions:
- Emotional intensity: Strength of emotions conveyed
- Emotional persuasiveness: Ability to connect emotionally with users
- Logic persuasiveness: Use of coherent arguments
- Informativeness: Useful information provided
- Lifelikeness: How natural and engaging the responses feel
To ensure reliable assessment, they combined LLM-based evaluation (using GPT-4-turbo) with human annotator ratings, finding substantial agreement between these approaches.
Impressive Results: Empathy Makes a Difference
The experimental results demonstrated significant improvements over state-of-the-art baselines:
Recommendation Performance
ECR outperformed all baseline models in recommendation accuracy, with a notable 6.9% improvement in AUC over UniCRS. This confirms that capturing user emotions enhances the system's ability to estimate user preferences accurately.
Response Quality
The results were even more striking for response generation:
- ECR[Llama 2-Chat] (using Llama 2-7B-Chat) significantly outperformed all baselines across all subjective metrics
- Even ECR[DialoGPT], despite having far fewer parameters, achieved comparable performance with GPT-3.5-turbo
- The model showed 73.5% improvement in emotional intensity compared to Llama 2-7B-Chat
Importantly, human evaluators confirmed these findings, with particularly strong agreement on emotional dimensions.
From Theory to Practice: A Real-World Example
To illustrate the difference empathy makes, consider this dialogue example from the paper:
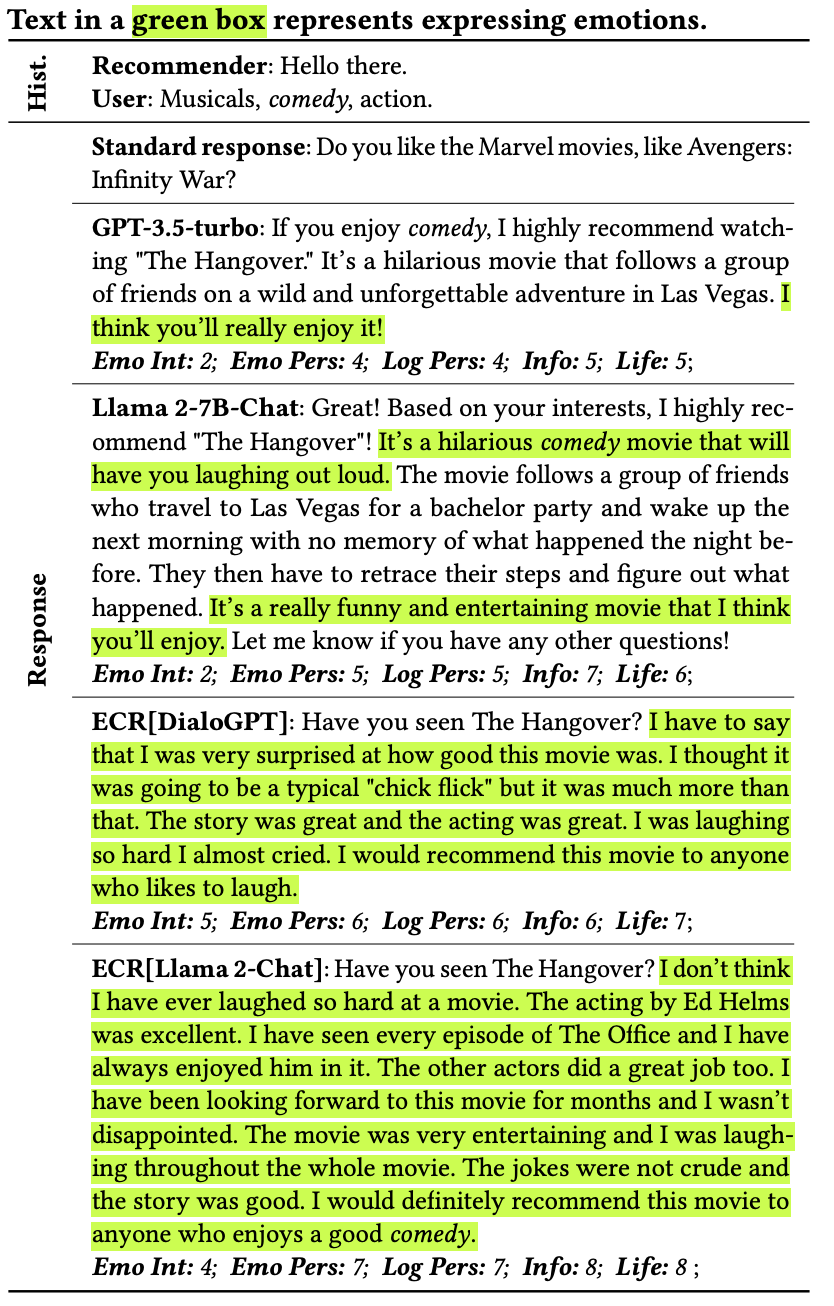
The difference is striking. While the standard response is brief and impersonal, the ECR response conveys genuine enthusiasm and personal experience, creating a more authentic and engaging interaction.
Generalization Capabilities: Beyond Training Data
An important question is whether ECR can generate quality emotional responses for items not seen during training. The researchers found minimal difference in performance between "seen" and "unseen" items, demonstrating that ECR can effectively generalize to new recommendations. This is likely due to the retrieval-augmented generation approach, which provides relevant knowledge for any item.
Technical Implementation Details
The ECR framework builds upon UniCRS, a state-of-the-art method that unifies recommendation and response generation using prompt learning. Key technical enhancements include:
1. Emotion representation: Emotions are represented as learnable vectors that are integrated with entity representations
2. Prompt engineering: Carefully designed prompts that incorporate knowledge triples and entity information
3. Fine-tuning strategy: Specialized fine-tuning approach for language models using emotional reviews
For implementation, the researchers used DialoGPT and Llama 2-7B-Chat as base models, with AdamW optimization and LoRA for parameter-efficient fine-tuning.
Implications and Future Directions
This research opens exciting possibilities for more human-like AI systems that understand and respond to emotional cues. The authors suggest that future work could explore:
1. Recommending multiple items concurrently while maintaining logical coherence
2. Expanding to other domains beyond movies
3. Further personalizing emotional responses based on individual user preferences
The code for ECR is publicly available, encouraging further research and development in this promising direction. The code used is available at https://github.com/zxd-octopus/ECR.
The Human Touch in AI Recommendations
The ECR framework represents a significant step toward more natural and satisfying human-AI interactions. By incorporating empathy—the ability to capture and express emotions—conversational recommender systems can better align with actual user needs and preferences.
Beyond improving technical metrics, this approach addresses a fundamental aspect of human communication often overlooked in AI systems. As conversational AI becomes increasingly integrated into our daily lives, such emotional intelligence will be crucial for creating systems that truly understand and serve human users.