As the digital landscape continues to evolve, search is moving beyond simple keyword matching to understanding the context and intent behind user queries. Personalized semantic search, powered by state-of-the-art artificial intelligence, embeddings, and machine learning technologies, is revolutionizing traditional keyword-based search, transforming the way we find and access information online. In this blog post, we will delve into the history and types of search, discuss the technical aspects, advantages, and disadvantages of each approach, and explore real-world examples of personalized semantic search in action, along with its impact on business metrics such as conversion, retention, and engagement.
What is Personalized Semantic Search?
Personalized semantic search is an advanced search technique that couples understanding the meaning, context, and intent behind user queries with personalization from past user behavior. By leveraging machine learning, artificial intelligence, and natural language processing technologies, personalized semantic search can deliver more accurate, relevant, and personalized search results, vastly improving the user experience. This approach enables search engines to move beyond mere keyword matching and offer a more intuitive and seamless information retrieval process.
One way personalized semantic search is often characterized is helping you “search the way you think”. Although you’re almost certainly using personalized semantic search already everyday in products from big tech companies, you can get a unique feel for what it’s like with search engines like You.com and Same.Energy.
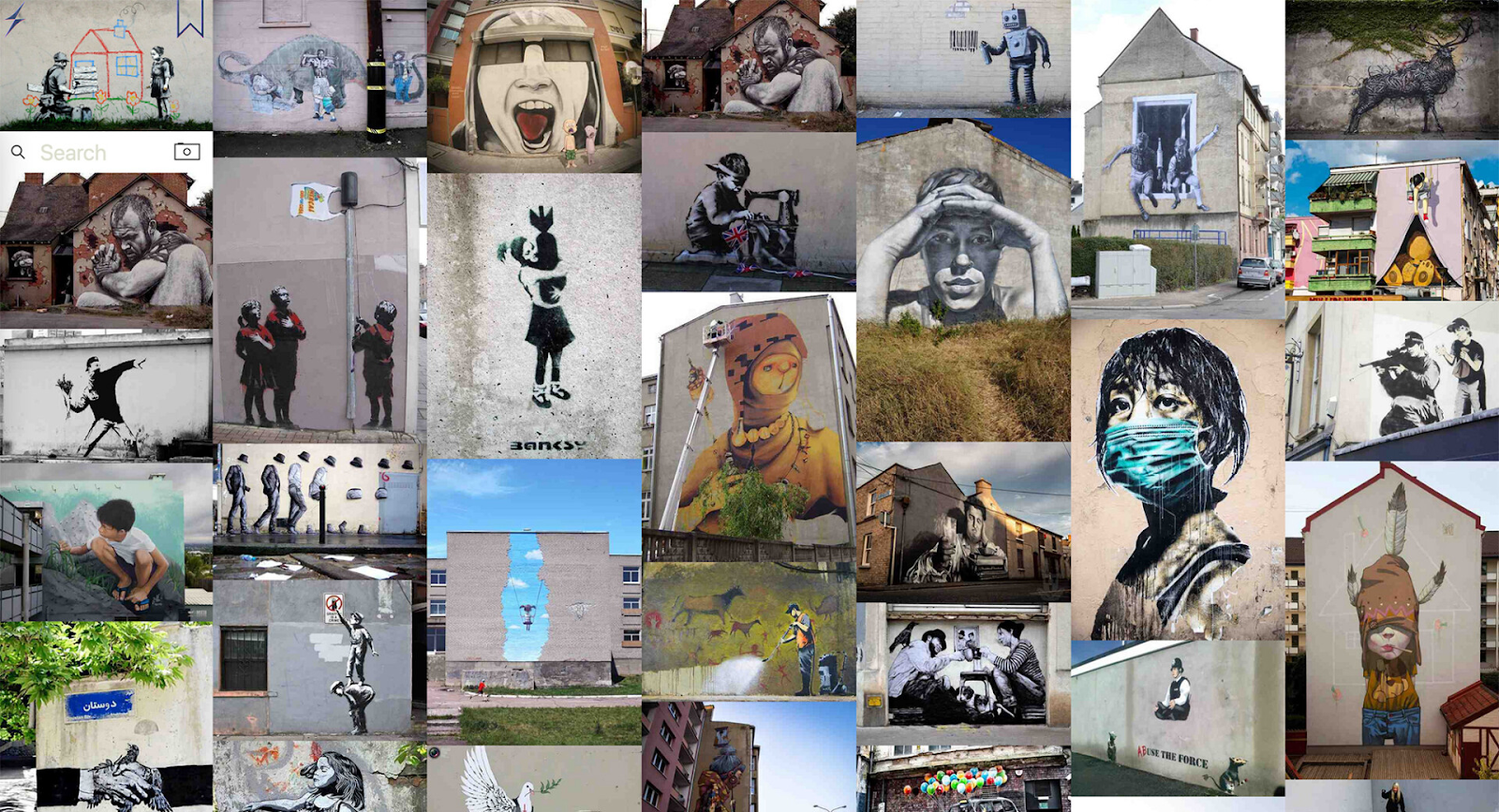
Keyword-based Search
Keyword-based search relies on explicitly matching keywords from user queries to the data with rules. These rules typically include factors like keyword frequency, location, and the presence of related terms.

Semantic Search
Building upon keyword-based search, semantic search employs AI, machine learning, and NLP to understand the meaning and context behind user queries, providing more accurate and relevant results. Techniques such as tokenization, part-of-speech tagging, and named entity recognition help analyze text, while machine learning algorithms identify patterns, draw connections, and make predictions based on the data.

Personalized Semantic Search
Personalized semantic search aim to balance the strengths and weaknesses of both approaches whilst taking into account past user behavior. It starts by conducting a keyword-based search to quickly identify potential matches. Then, it incorporates semantic analysis techniques to better understand the meaning and context of the user's query and the content of the documents. Finally it personalizes the results by re-ranking them based on past user behavior. This enables the search system to refine and rank the search results more accurately, consider the presence of keywords, the semantic relationships between the words and user interests.
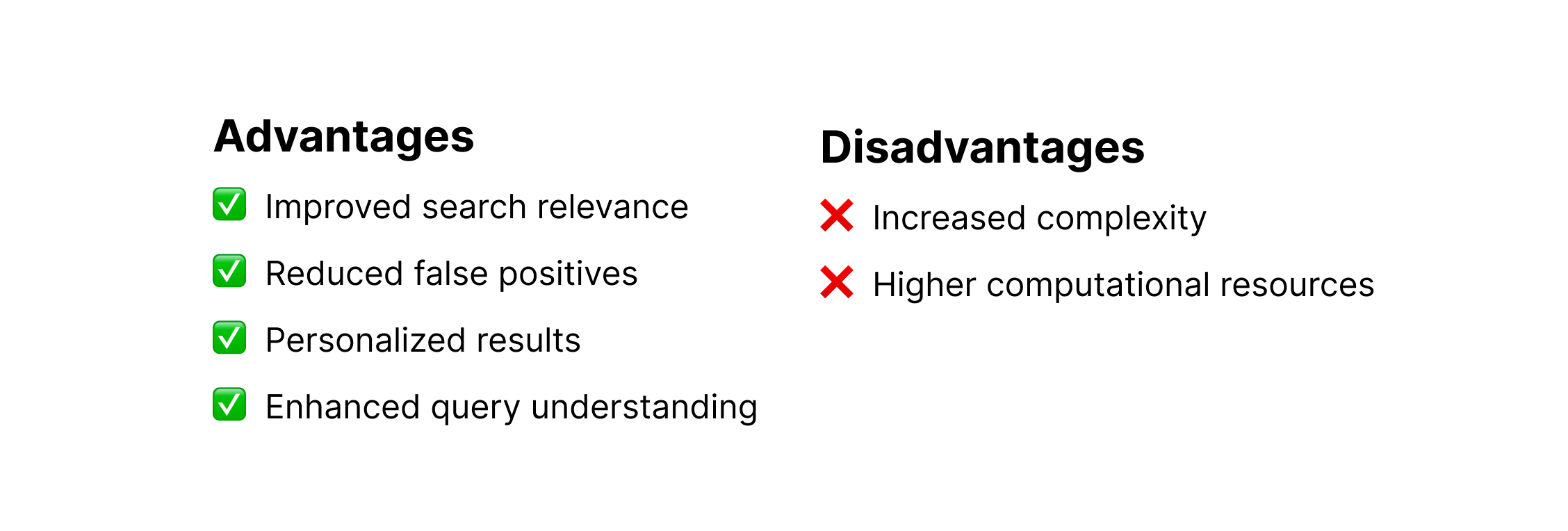
Real-World Examples of Personalized Semantic Search
Amazon
The e-commerce giant has implemented semantic search to improve the accuracy and relevance of search results, leading to increased conversion rates and customer retention. Semantic search enables shoppers to find products more easily and delivers personalized recommendations, enhancing the overall shopping experience.
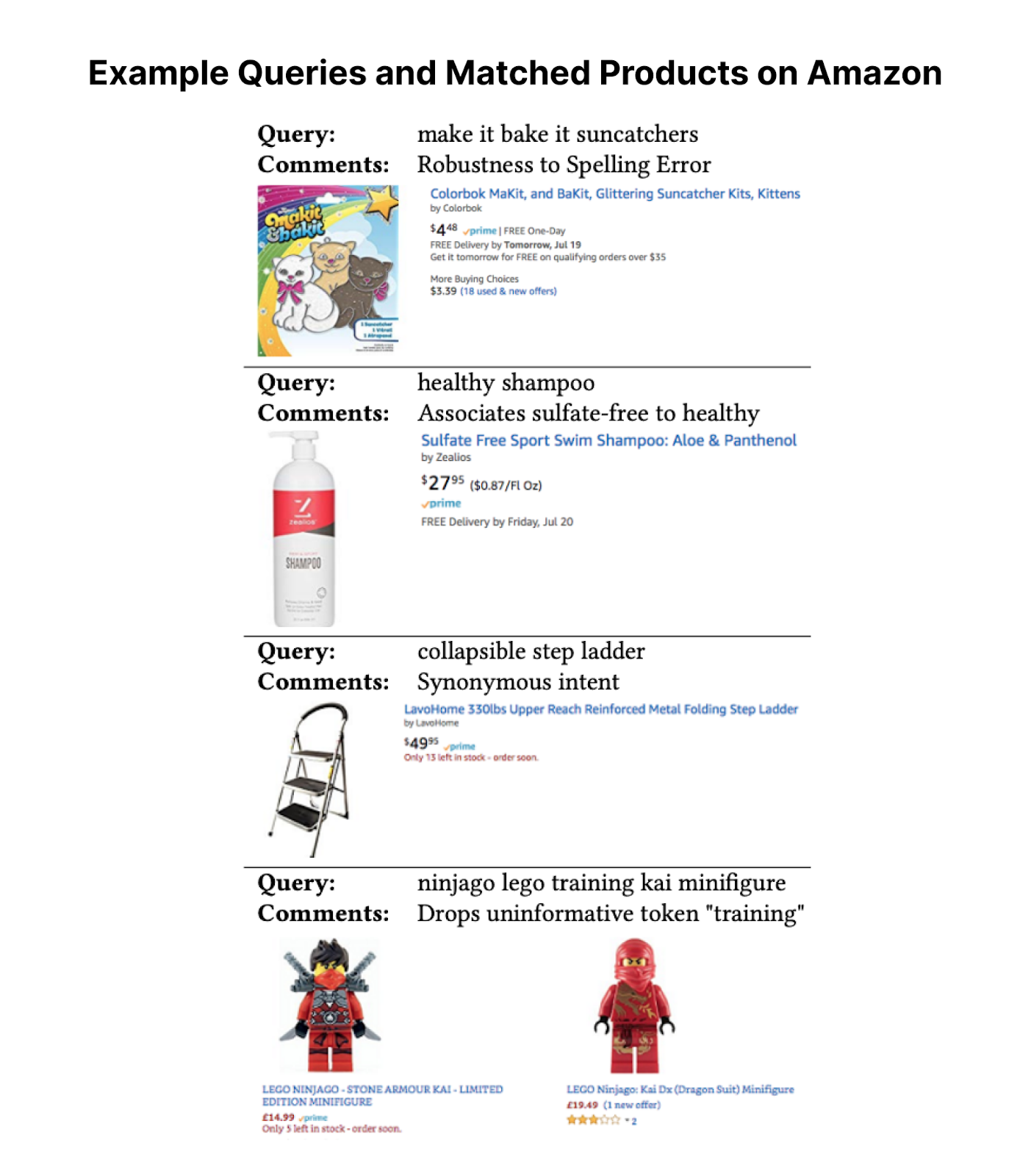
Spotify
By leveraging AI-powered semantic search and embeddings, Spotify can better understand user preferences and provide better results for artists, podcasts and songs even if many of the keywords in the search query are not present.

eBay
By adopting semantic search, eBay has significantly improved the relevance and accuracy of search results, leading to higher conversion rates and a better shopping experience for users.
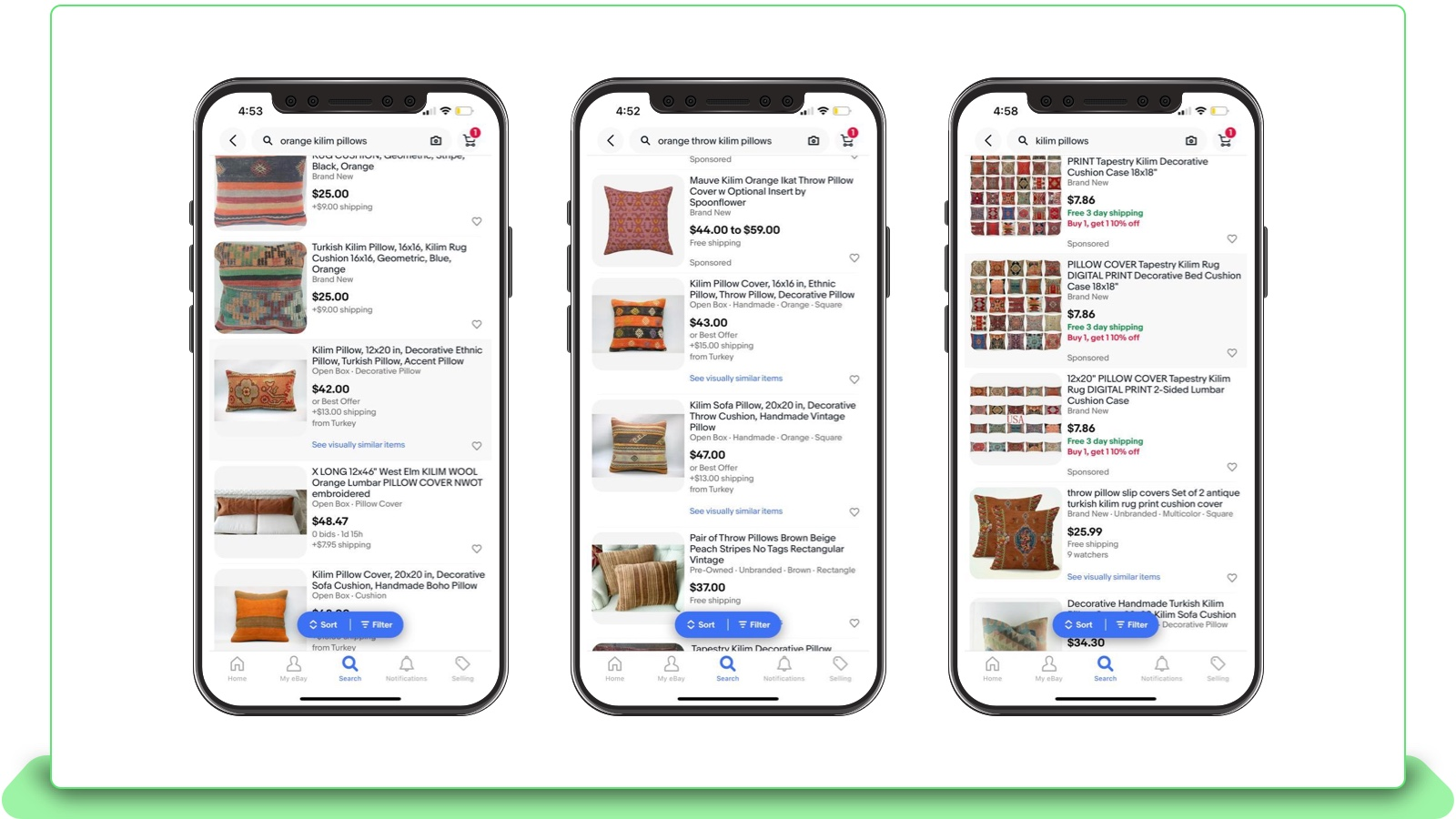
The Role of AI, Embeddings, and Large Language Models in Search and Recommendation Systems
The advancements in artificial intelligence, embeddings, and large language models have been instrumental in the evolution of search and recommendation systems. Big tech companies like Amazon, Spotify, Facebook, eBay, and TikTok have moved away from traditional keyword-based search to AI-powered personalized semantic search, utilizing advanced technologies like transformers and embeddings to analyze and understand user queries.
- AI and Machine Learning: Machine learning algorithms play a critical role in semantic search by identifying patterns, drawing connections, and making predictions based on the available data. AI helps search understand the context and intent behind queries, leading to more accurate and relevant results.

- Embeddings: Embeddings are mathematical representations of words and phrases that capture their meaning and relationships within a high-dimensional vector space. These embeddings enable us to understand the semantic relationships between words, phrases, and documents, leading to improved search results.
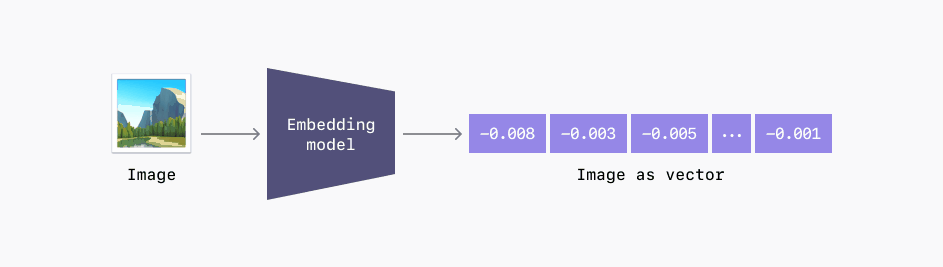
- Large Language Models: Models like GPT-4, BERT and LLaMa and transformers have revolutionized the way we can process and analyze text data. These models generate highly contextualized embeddings to understand complex language patterns. They are being used in various forms today to enable search to provide more accurate, relevant, and personalized results.

Conclusion
The advent of personalized semantic search is transforming the way we access and retrieve information. By leveraging new AI technologies such as embeddings, and large language models, personalized semantic search transcends the limitations of traditional keyword-based search, providing users with a more accurate, relevant, and personalized search experience. As the technology continues to evolve, it is evident that incorporating a personalized semantic approach for search and ranking will become the default way we discover online. If you'd like to explore this at your company enter your email below.