The AI-Native Personalization Platform
Shaped helps you rapidly experiment with AI search, recommendations, and personalization. Understand and control your growth with a real-time, configurable relevance engine built for scale.
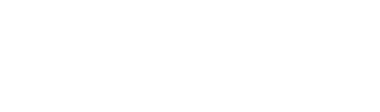
Why Shaped
Easy Set-Up
Connect and deploy rapidly with direct integration to your existing data sources.
Real-Time Adaptability
Ingest and re-rank in real-time using behavioral signals.
State-of-the-Art Model Library
Fine tune LLMs and neural ranking models for state-of-the-art performance.
Highly Customizable
Build and experiment with ranking and retrieval components for any use case.
Explainable Results
Visualize, evaluate, and interpret your data with in-session analytics and performance metrics.
Secure Infrastructure
Scale with enterprise-grade security that’s GDPR and SOC2 compliant.

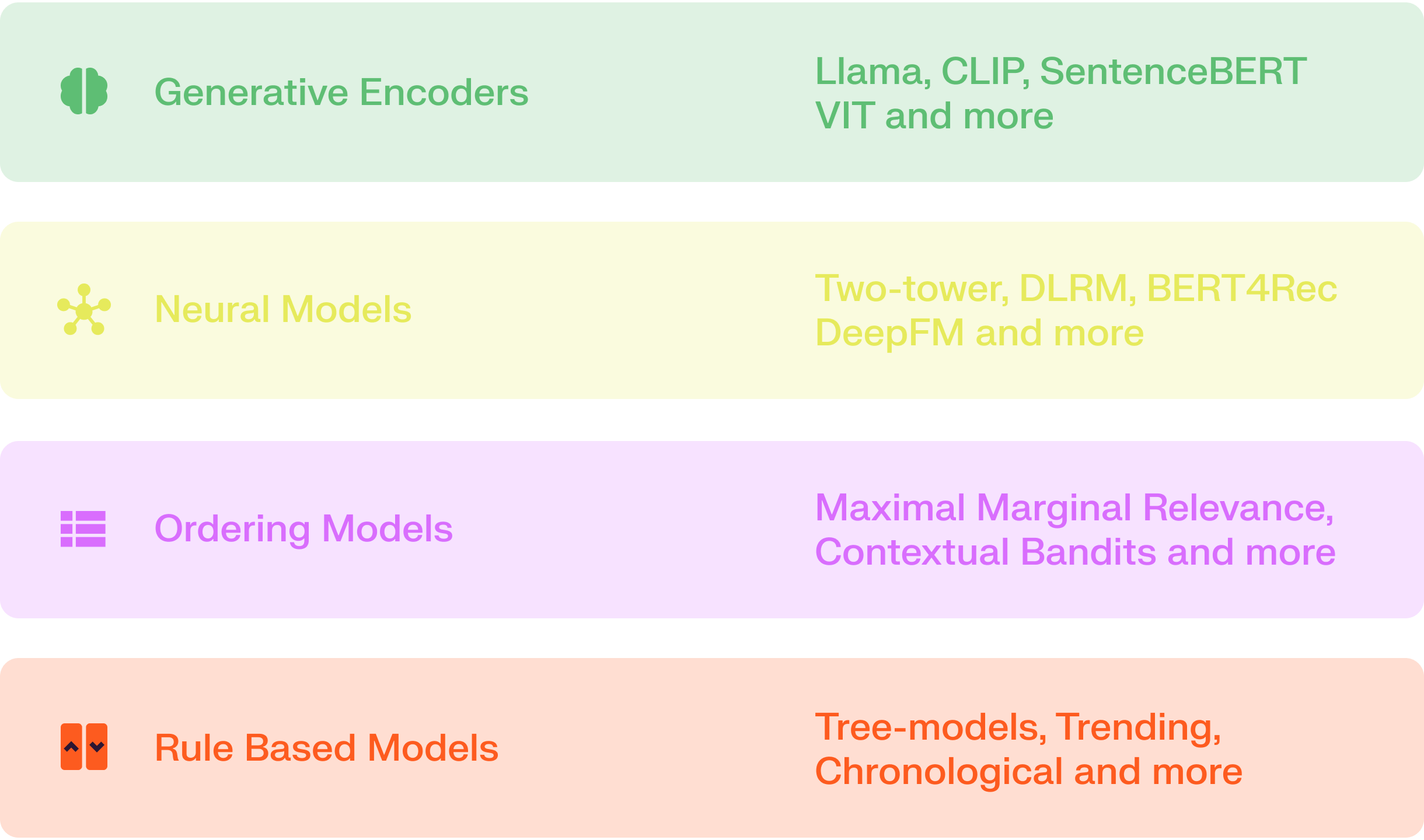
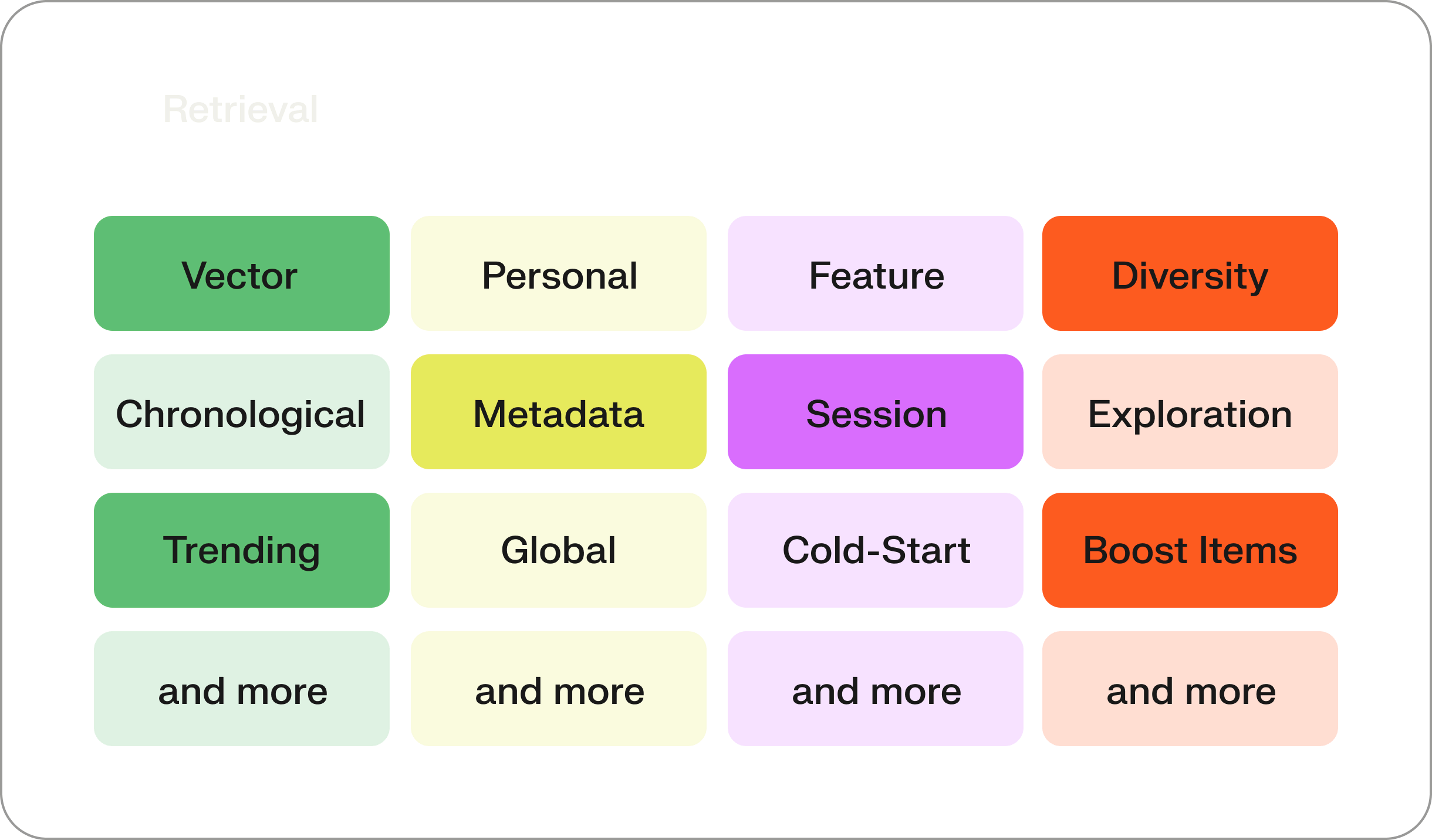


Shaped Overview
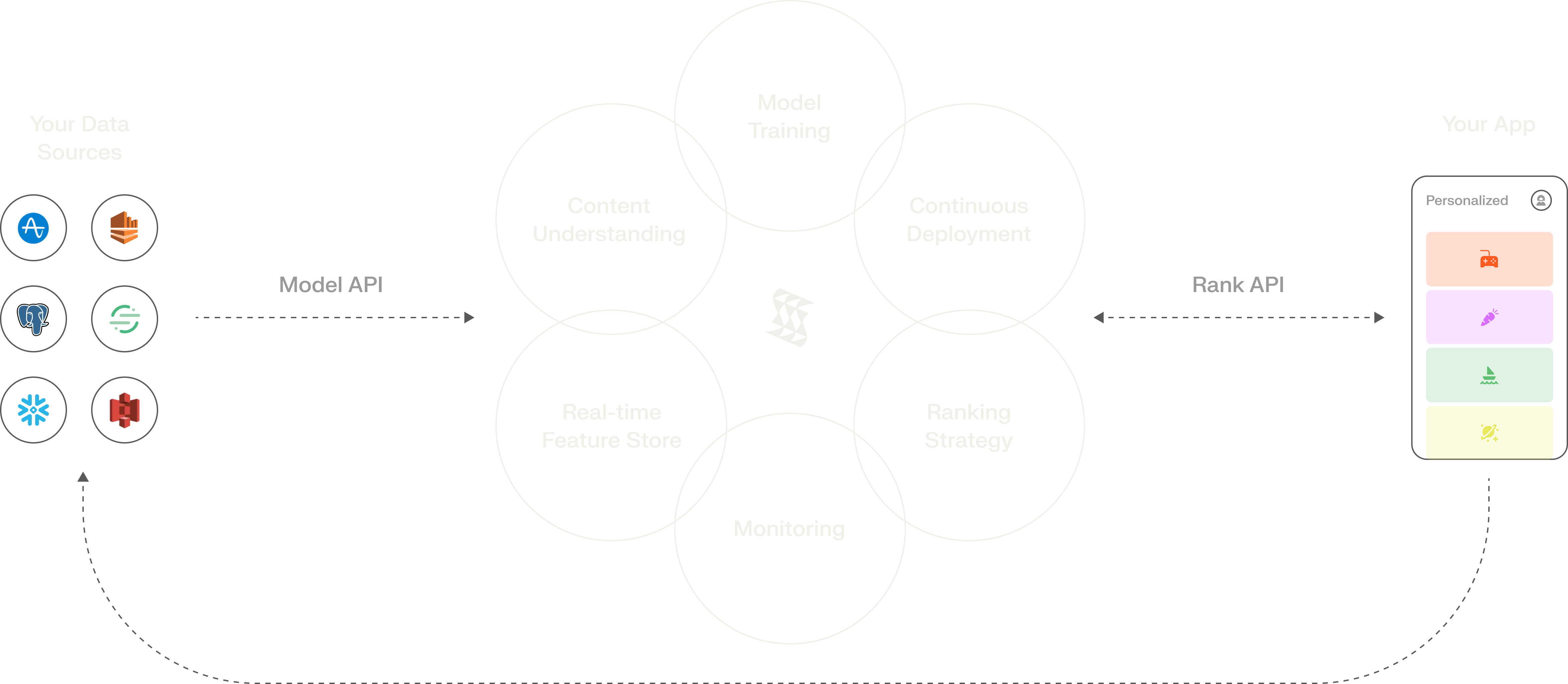

Designed for technical teams
Whether you’re an expert in recommendation systems, a casual machine-learning practitioner or a novice developer, Shaped is built for you.
await rank({model_name: 'for_you_feed', user_id: '3'});
client = shaped.Client(api_key='your_api_key') client.rank('for_you_feed', user_id=3, limit=2)
"ids": [
"carrot",
"apple"
],
"scores": [
0.919,
0.832
]
}
Solutions for every platform
Marketplaces
Optimize all sides of any marketplace. Improve every step of a buyers’ and sellers’ experience.
Social Media
Turn traffic into community. Boost engagement and retention.
Media Platforms
Turn traffic into subscriptions. Boost revenue and engagement.
E-Commerce
Turn traffic into conversions. Boost engagement and retention.
Get up and running with one engineer in one sprint
Guaranteed lift within your first 30 days or your money back
The integration process is quick. You can connect your data to Shaped in minutes, train your first model in hours, and fully integrate into your app in days.
While all three aim to improve relevance and personalization, Shaped is built as a unified, AI-native platform designed for real-time, deeply customizable search and recommendation systems—purpose-built for modern data teams.
- Unified search & recommendations
Shaped merges search and recommendations into a single system, enabling cross-learning between the two. Algolia treats them as separate products with siloed logic, and AWS Personalize doesn’t support search at all, requiring teams to stitch together separate tools. - True AI-native foundation
Shaped was built from the ground up for machine learning. It uses transformer models, multi-objective optimization, and real-time learning loops to adapt quickly to user behavior. Algolia and AWS Personalize offer AI-enhanced capabilities, but their core systems weren’t originally designed for modern ML workflows, which limits depth and flexibility. - Designed for ML engineers, not just developers
Shaped integrates directly with data warehouses, supports SQL-based feature engineering, and exposes model internals. It’s a tool technical teams can fully control and extend. Algolia abstracts most of the logic behind APIs, and AWS Personalize uses pre-packaged “recipes” with limited visibility and customization. - Experimentation and iteration at the core
Shaped supports rapid experimentation with ranking strategies, feature sets, and objective functions—crucial for teams optimizing for business-specific KPIs. Algolia’s experimentation is surface-level, and AWS Personalize’s workflow makes iterative testing slower and more infrastructure-heavy. - Cold-start & multi-modal understanding
Shaped uses rich embeddings from text, images, and behavioral data to deliver relevance even with sparse signals. This gives it an edge in cold-start scenarios and unstructured data. Algolia is optimized for keyword-based relevance, and AWS Personalize depends heavily on structured historical interactions.
Shaped isn’t just another tool in the stack—it’s an AI-native foundation for delivering relevance across your entire product.
Certainly! Shaped is designed to be used for all of your ranking use-cases. Typically the companies we work with deploy dozens. Once your data is connected to Shaped creating additional ranking models is easy. See our docs for more information.
There is no minimum amount of data required. Collecting interactions, for example clicks, views and or impressions is the only requirement.
For more information see this blog post How much data do I need for a recommendation system?
The high cost of hiring multiple machine-learning engineers, the long time required to build and the on-going full-time maintenance required. We handle scalability and reliability without the worries. Shaped can take you from 0 to 1 in a few days at a fraction of the cost.
Our pricing is flat-fee monthly determined by usage. Once we understand your approximate number of monthly active users, items counts, and particular implementation details, we’ll be able to provide a pricing estimate.
Shaped was built from the ground-up with security as a top priority. We operate as a cloud software-as-a-service (SaaS) platform, and only retrieve from your connected datastores when necessary to build your ranking algorithms. After training, most of your data is discarded, other than specific non-identifiable encoded features that are used at inference. We only require read access to your datasets and customer data never has to be persisted within Shaped. Furthermore, Shaped works on encrypted data if you need an added layer of security.
Shaped uses best physical, virtual, network and operational security practices. We rely on role-based authentication for all data access and records audit logs for every action. We avoid data replication and have clear multi-tenant isolation policies to ensure sensitive and critical systems are separated. Shaped uses isolated VPCs for all production deployments. Data is encrypted at rest using AES-256 encryption or higher and all ingress and egress traffic is encrypted via TLS 1.2+.
For more information see here.